Securing Generative AI: Best Practices for Attack Surface Management
AZ
Understanding the Importance of Securing Generative AI
Generative AI is transforming industries with its ability to create content, simulate scenarios, and automate complex tasks. However, with these capabilities comes an increased attack surface that needs vigilant management. As we integrate AI into more systems, understanding and securing this technology becomes crucial to prevent potential vulnerabilities from being exploited.
Ensuring the security of generative AI systems involves several layers of protection, from data input to deployment. These systems rely on vast amounts of data, making them attractive targets for malicious actors. By implementing effective attack surface management (ASM), organizations can safeguard their AI assets and maintain robust cybersecurity defenses.
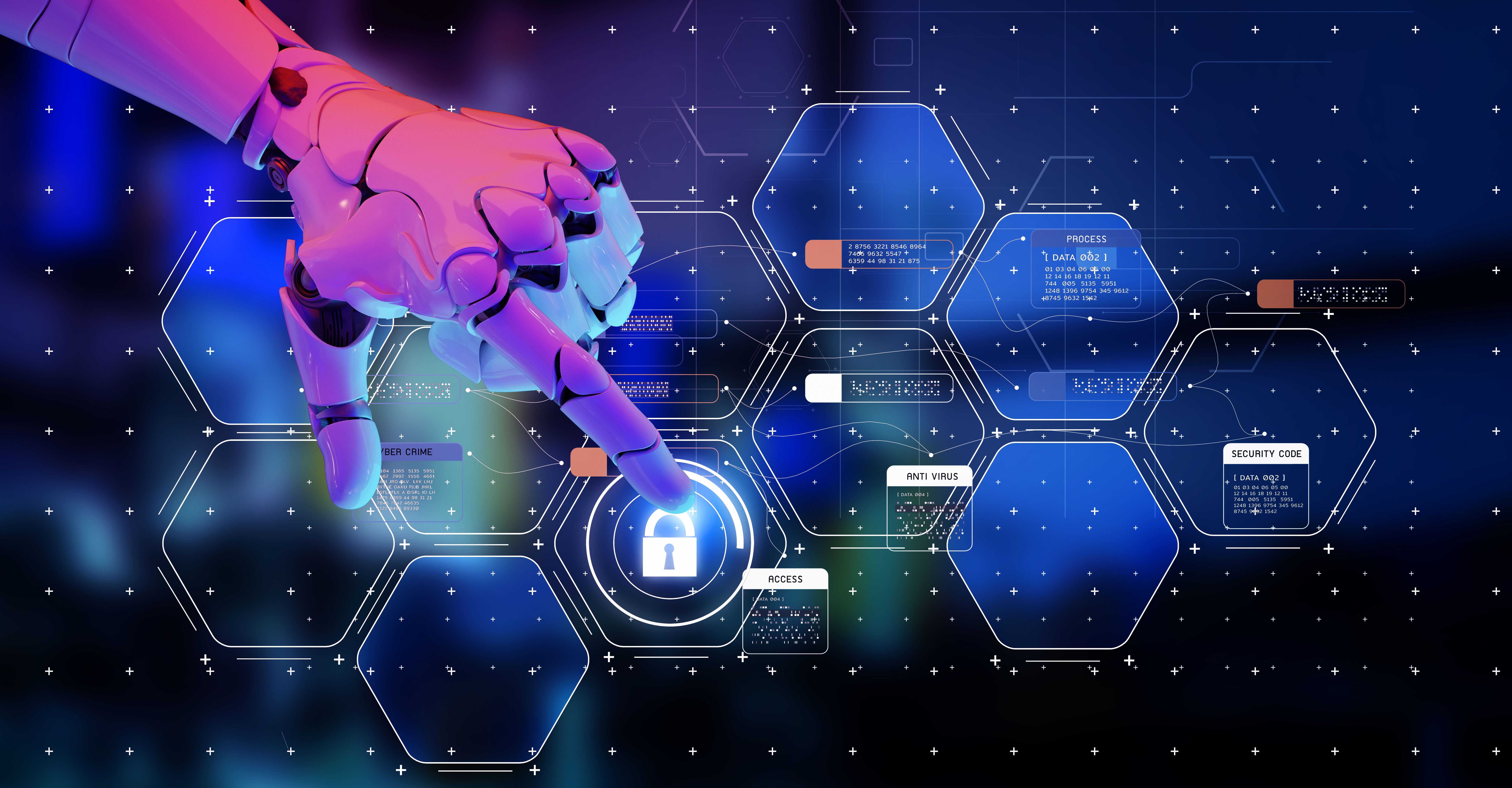
Identifying and Assessing Vulnerabilities
The first step in securing generative AI is identifying potential vulnerabilities within your system. This involves conducting a comprehensive risk assessment to understand where weaknesses may lie. Consider both external threats and internal errors that could lead to security breaches.
Vulnerabilities in generative AI can stem from various sources, including data poisoning, model theft, and adversarial attacks. Recognizing these risks allows organizations to develop targeted strategies to mitigate them, ensuring that the AI system remains robust and reliable.
Implementing Best Practices in ASM
Effective attack surface management for generative AI requires a multi-faceted approach. Here are some best practices organizations should consider:
- Regular Monitoring: Continuously monitor AI systems to detect unusual activities that could indicate a security breach. This proactive approach helps in early detection and response.
- Access Controls: Limit access to the AI system to authorized personnel only. Implement role-based access controls to ensure users have the minimum necessary permissions.
- Data Encryption: Use encryption techniques to protect data at rest and in transit, reducing the risk of unauthorized access.
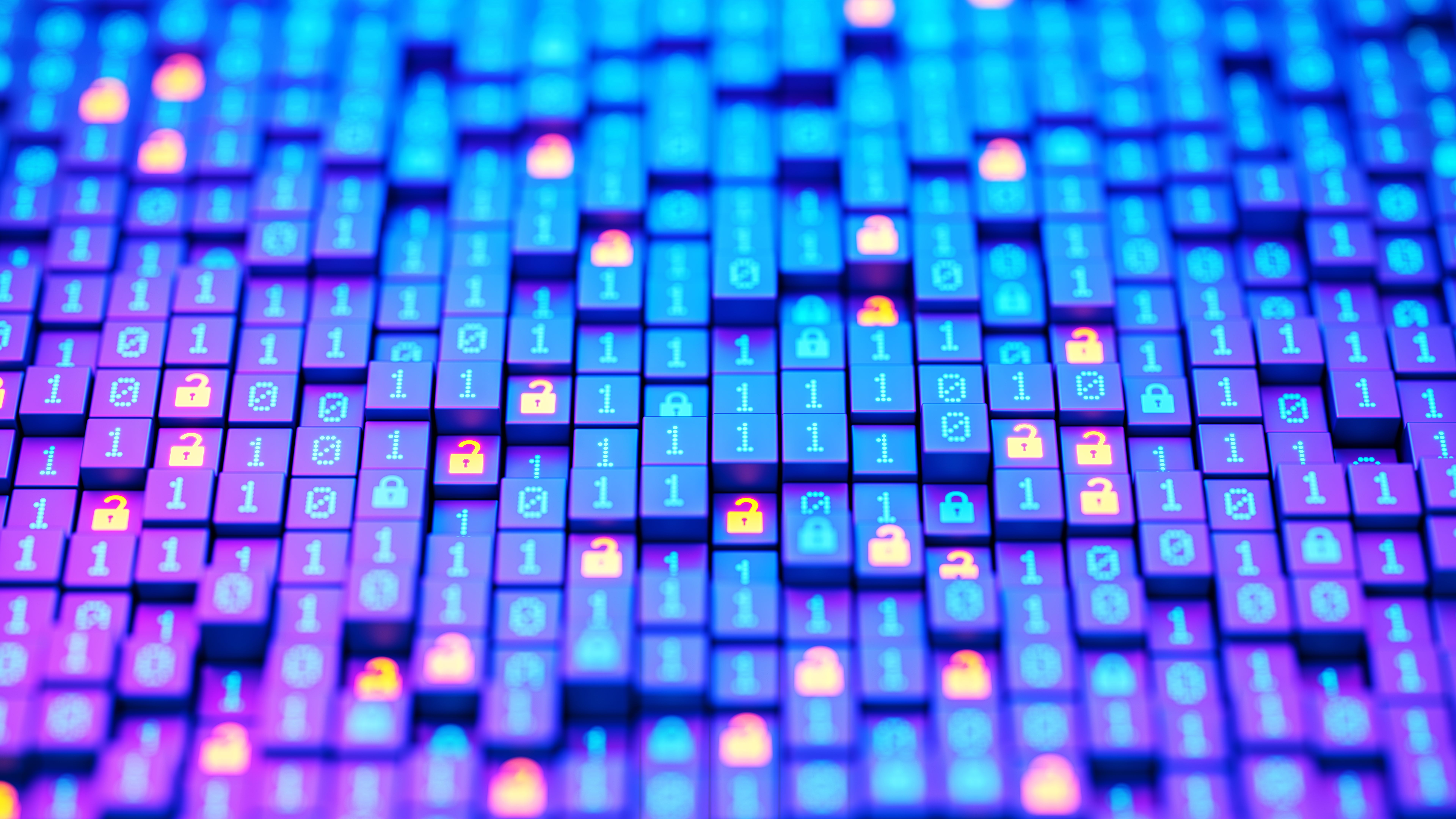
Training and Awareness for Stakeholders
Another critical aspect of securing generative AI involves training stakeholders about potential risks and the importance of adhering to security protocols. Organizations should conduct regular workshops and training sessions to educate employees on security best practices.
An informed workforce is a powerful defense against potential security threats. By fostering a culture of cybersecurity awareness, organizations can better protect their generative AI systems from human error or insider threats.
Embracing Advanced Security Technologies
As generative AI evolves, so do the technologies designed to protect it. Advanced tools such as anomaly detection systems and machine learning-based threat intelligence can enhance traditional security measures.
These technologies provide an additional layer of protection by automatically identifying patterns that deviate from normal behavior, allowing for rapid response to potential threats.
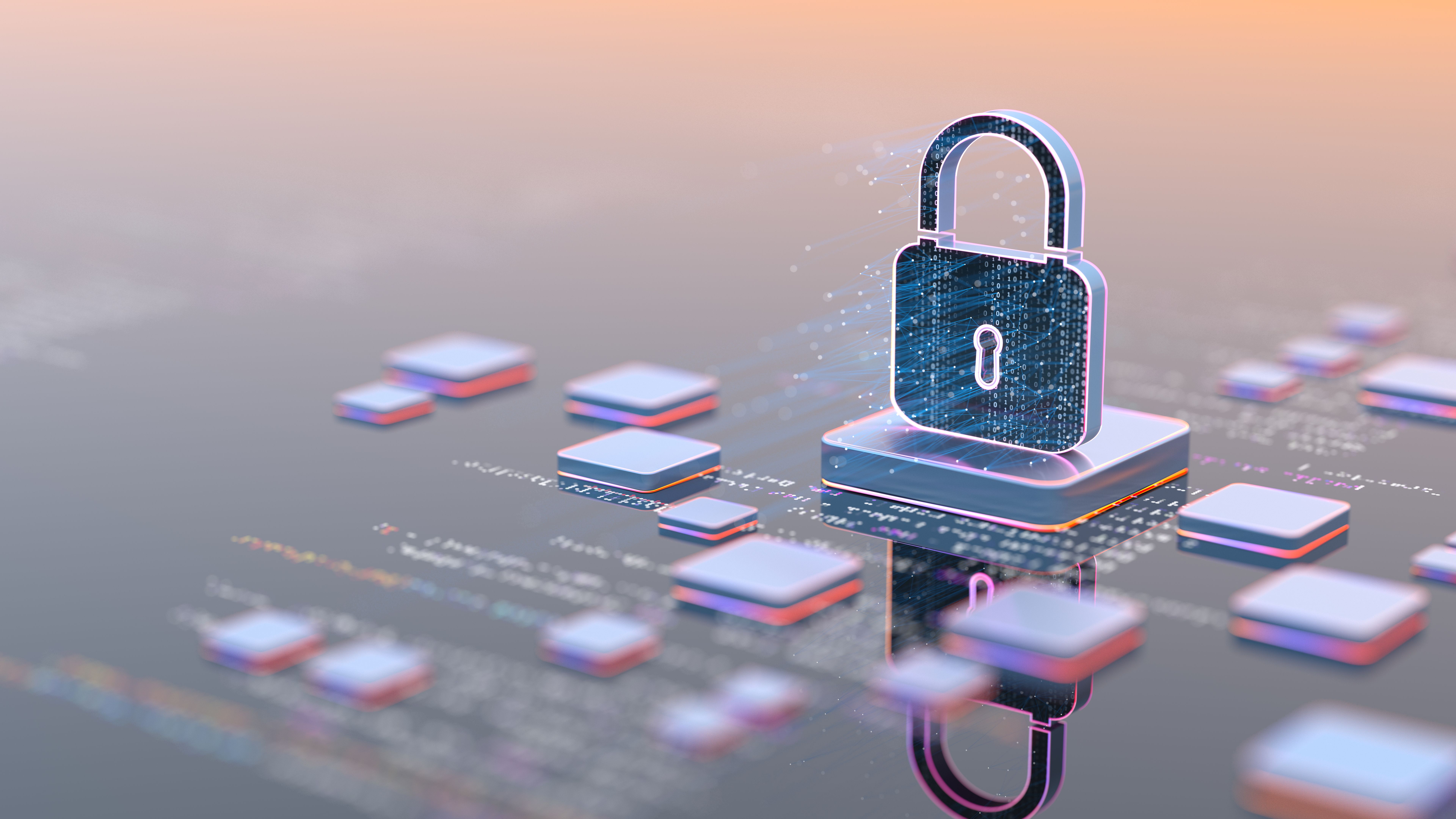
Regularly Updating and Patching Systems
Keeping AI systems updated is essential in safeguarding against emerging threats. Regularly patching software and hardware components closes known vulnerabilities that attackers could exploit.
This practice ensures that AI models operate with the latest security features and protections, maintaining the integrity of the system over time.
The Future of Secure Generative AI
The landscape of generative AI is rapidly evolving, bringing with it new opportunities and challenges. As organizations continue to adopt this technology, prioritizing security through effective attack surface management will remain a crucial task.
By implementing these best practices and staying informed about emerging threats, organizations can harness the power of generative AI while minimizing risks, ensuring a secure and resilient technological future.