Understanding AI Security Taxonomy: A Comprehensive Guide
AZ
Introduction to AI Security Taxonomy
Artificial Intelligence (AI) has rapidly become an integral part of various sectors, from healthcare to finance. With its widespread adoption, understanding AI security is crucial to protect sensitive data and maintain trust in AI systems. AI security taxonomy provides a structured framework for identifying and addressing potential security threats associated with AI technologies.
In this comprehensive guide, we will delve into the different components of AI security taxonomy, explore the challenges it faces, and discuss best practices to ensure robust security measures are in place.
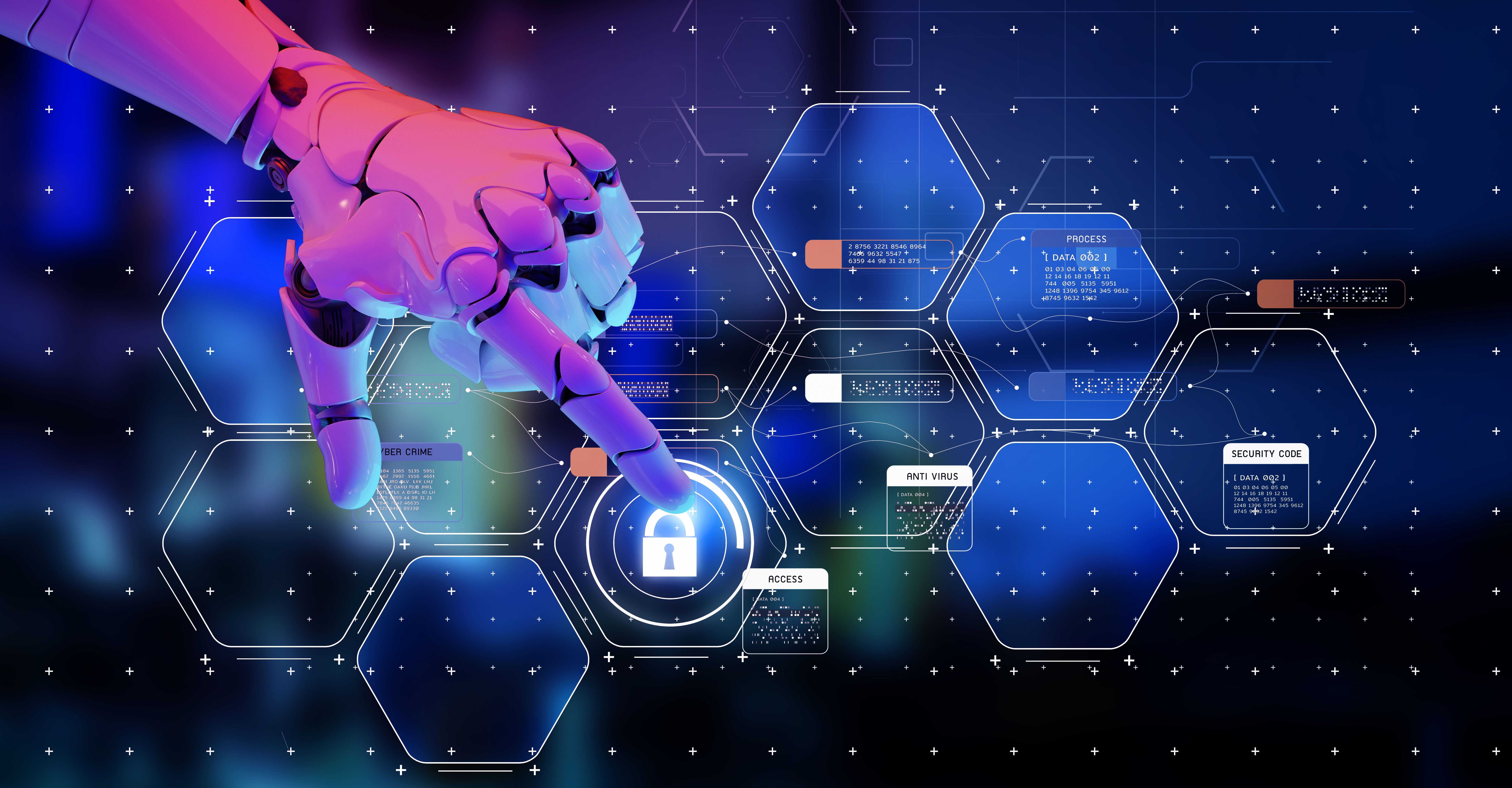
Components of AI Security Taxonomy
Data Security
Data security is a fundamental aspect of AI security taxonomy. It involves protecting data from unauthorized access and ensuring its integrity. With AI systems relying heavily on vast amounts of data, safeguarding this information is paramount. Data security measures include encryption, access controls, and data anonymization.
Model Security
Model security focuses on protecting the AI models themselves from threats such as adversarial attacks. These attacks can manipulate models to produce incorrect outputs, causing potential harm. Techniques like adversarial training and robust model architectures are employed to enhance model security.
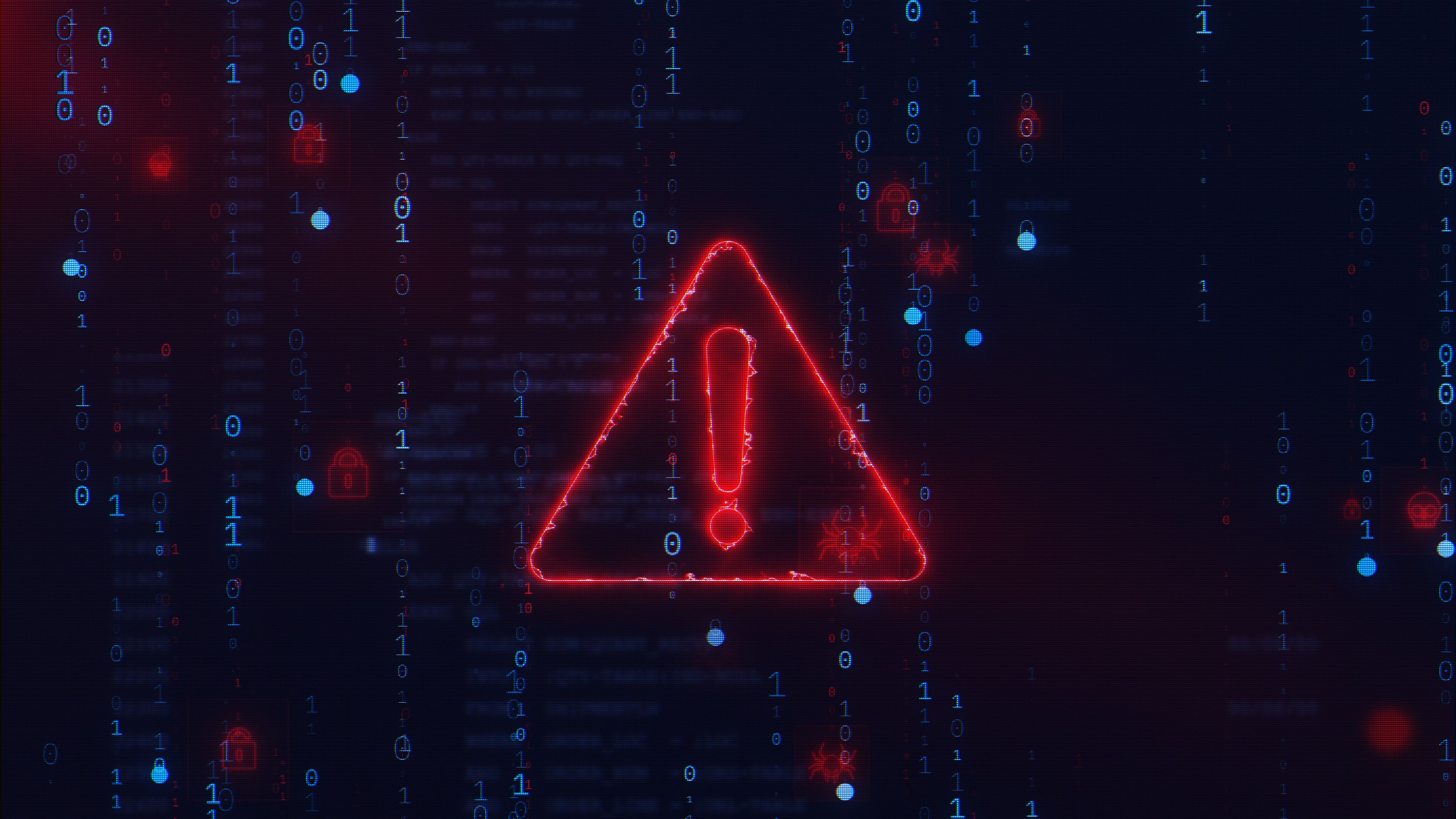
Challenges in AI Security
Adversarial Attacks
One of the significant challenges in AI security is adversarial attacks. These attacks involve subtly altering inputs to deceive machine learning models. They can lead to erroneous decisions in critical applications like autonomous vehicles or healthcare diagnostics. Understanding and mitigating adversarial attacks is a key focus area in AI security research.
Data Privacy Concerns
AI systems often require large datasets, which may contain sensitive information. Ensuring data privacy while maintaining the utility of the dataset is a delicate balance. Techniques such as differential privacy and federated learning are being developed to address these concerns without compromising model performance.
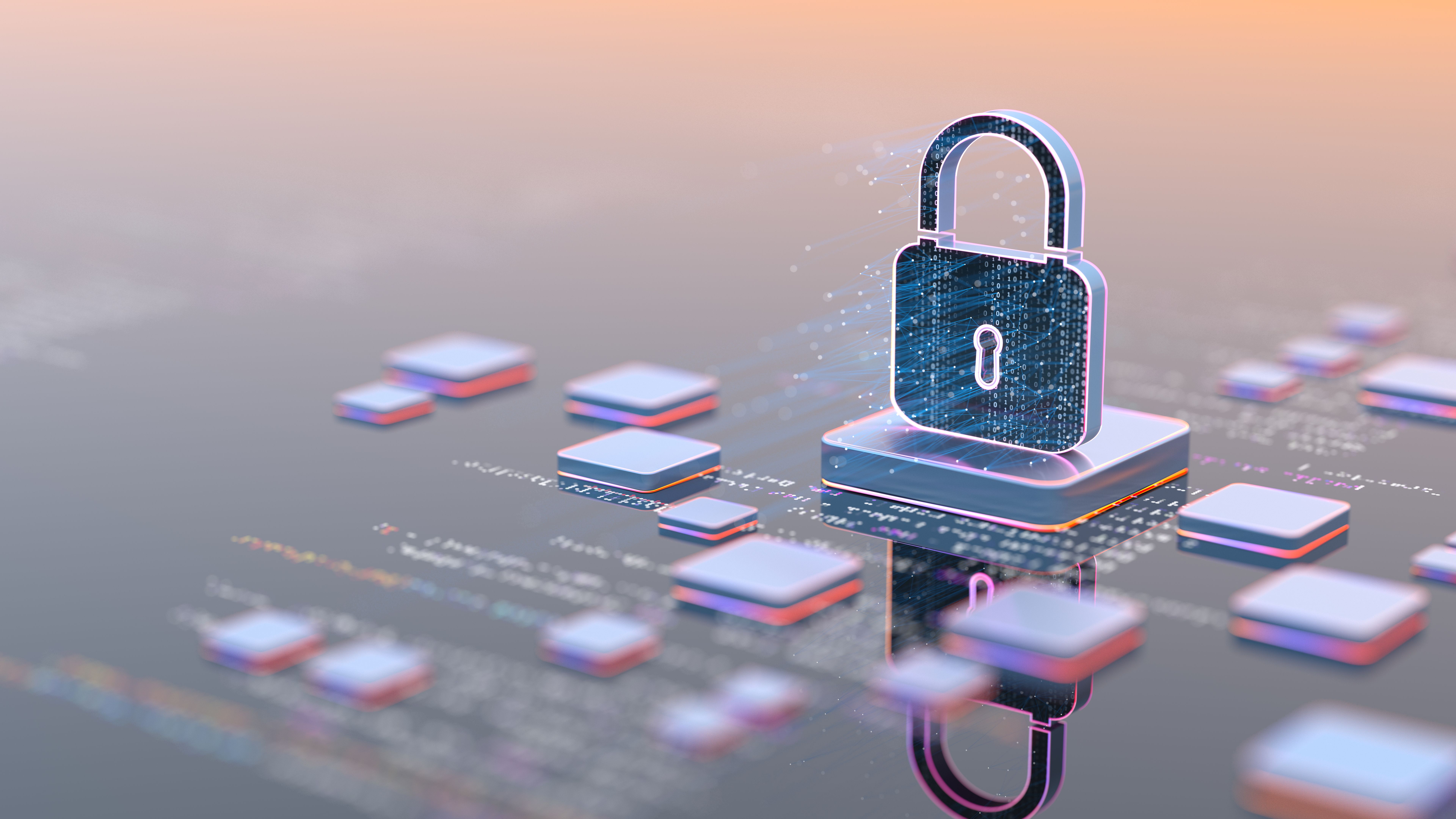
Best Practices for Enhancing AI Security
Regular Security Audits
Conducting regular security audits is essential to identify vulnerabilities in AI systems. These audits help organizations stay ahead of potential threats by evaluating their current security measures and implementing necessary improvements.
Implementing Robust Access Controls
Access controls are crucial for preventing unauthorized access to AI systems and data. By implementing multi-factor authentication and role-based access controls, organizations can safeguard their AI resources from malicious actors.
- Multi-factor Authentication
- Role-based Access Controls
- Encryption of Sensitive Data
The Future of AI Security
The field of AI security is continually evolving as new threats emerge and technologies advance. Researchers are actively developing innovative solutions to enhance the resilience of AI systems against cyber threats. Collaboration between academia, industry, and government is essential to create a secure AI ecosystem that benefits everyone.
As AI continues to permeate every aspect of our lives, understanding and implementing effective AI security measures will be critical to ensuring safe and reliable systems.
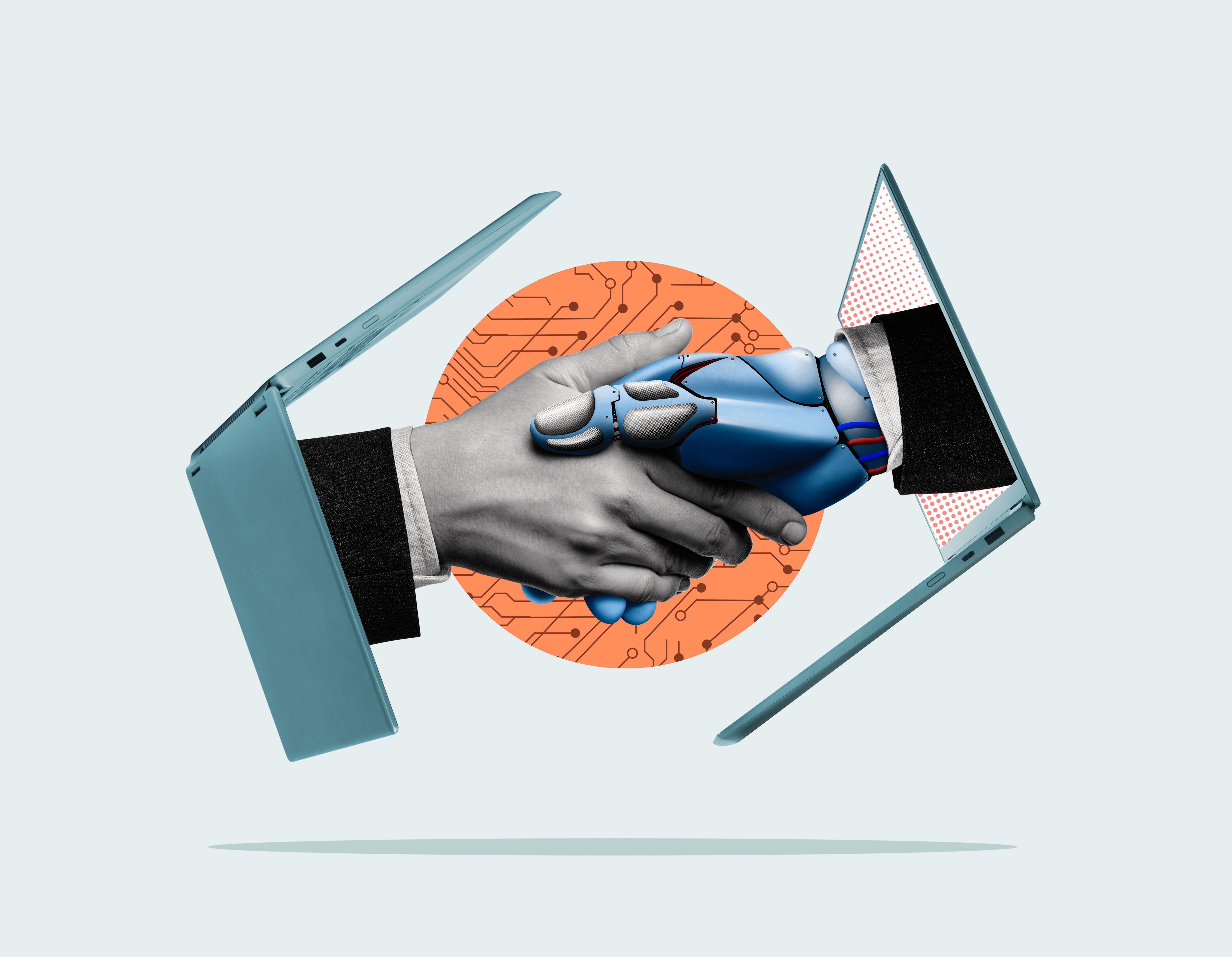